Kubeflow vs MLFlow: which one to choose?
Andreea Munteanu
on 23 June 2023
Data scientists and machine learning engineers are often looking for tools that could ease their work. Kubeflow and MLFlow are two of the most popular open-source tools in the machine learning operations (MLOps) space. They are often considered when kickstarting a new AI/ML initiative, so comparisons between them are not surprising.
This blog covers a very controversial topic, answering a question that many people from the industry have: Kubeflow vs MLFlow: Which one is better?
Both products have powerful capabilities but their initial goal was very different. Kubeflow was designed as a tool for AI at scale, and MLFlow for experiment tracking. In this article, you will learn about the two solutions, including the similarities, differences, benefits and how to choose between them.

What is Kubeflow?
Kubeflow is an open-source end-to-end MLOps platform started by Google a couple of years ago. It runs on any CNCF-compliant Kubernetes and enables professionals to develop and deploy machine learning models. Kubeflow is a suite of tools that automates machine learning workflows, in a portable, reproducible and scalable manner.
Kubeflow gives a platform to perform MLOps practices, providing tooling to:
- spin up a notebook
- do data preparation
- build pipelines to automate the entire ML process
- perform AutoML and training on top of Kubernetes.
- serve machine learning models using Kserve
Kubeflow added KServe to the default bundle, offering a wide range of serving frameworks, such as NVIDIA Triton Inference Server are available. Whether you use Tensorflow, PyTorch, or PaddlePaddle, Kubeflow enables you to identify the best suite of parameters for getting the best model performance. Kubeflow has an end-to-end approach to handling machine learning processes on Kubernetes. It provides capabilities that help big teams also work proficiently together, using concepts like namespace isolation.
Charmed Kubeflow is Canonical’s official distribution. Charmed Kubeflow facilitates faster project delivery, enables reproducibility and uses the hardware at its fullest potential. With the ability to run on any cloud, the MLOps platform is compatible with both public clouds, such as AWS or Azure, as well as private clouds. Furthermore, it is compatible with legacy HPC clusters, as well as high-end AI-dedicated hardware, such as NVIDIA’s GPUs or DGX. Charmed Kubeflow benefits from a wide range of integrations with various tools such as Prometheus and Grafana, as part of Canonical Observability Stack, Spark or NVIDIA Triton. It is a modular solution that can decompose into different applications, such that professionals can run AI at scale or at the edge.
What is MLFlow?
MLFlow is an open-source platform, started by DataBricks a couple of years ago. It is used for managing machine learning workflows. It has various functions, such as experiment tracking. MLFlow can be integrated within any existing MLOps process, but it can also be used to build new ones. It provides standardised packaging, to be able to reuse the models in different environments. However, the most important part is the model registry component, which can be used with different ML tools. It provides guidance on how to use machine learning workloads, without being an opinionated tool that constrains users in any manner.

Charmed MLFlow is Canonical’s distribution of MLFlow. At the moment, it is available in Beta. We welcome all data scientists, machine learning engineers or AI enthusiasts to try it out and share feedback. It is a chance to become an open source contributor while simplifying your work in the industry.
Kubeflow vs MLFlow
Both Kubeflow and MLFlow are open source solutions designed for the machine learning landscape. They received massive support from industry leaders, as well as a striving community whose contributions are making a difference in the development of the project. The main purpose of Kubeflow and MLFlow is to create a collaborative environment for data scientists and machine learning engineers, to develop and deploy machine learning models in a scalable, portable and reproducible manner.
However, comparing Kubeflow and MLFlow is like comparing apples to oranges. From the very beginning, they were designed for different purposes. The projects evolved over time and now have overlapping features. But most importantly, they have different strengths. On one hand, Kubeflow is proficient when it comes to machine learning workflow automation, using pipelines, as well as model development. On the other hand, MLFlow is great for experiment tracking and model registry. Also, from a user perspective, MLFlow requires fewer resources and is easier to deploy and use by beginners, whereas Kubeflow is a heavier solution, ideal for scaling up machine learning projects.
Overall, Kubeflow and MLFlow should not be compared on a one-to-one basis. Kubeflow allows users to use Kubernetes for machine learning in a proper way and MLFlow is an agnostic platform that can be used with anything, from VSCode to JupyterLab, from SageMake to Kubeflow. The best way, if the layer underneath is Kubernetes, is to integrate Kubeflow and MLFlow and use them together. Charmed Kubeflow and Charmed MLFlow, for instance, are integrated, providing the best of both worlds. The process of getting them together is easy and smooth since we already prepared a guide for you.
Kubeflow vs MLFlow: which one is right for you?
Follow our guide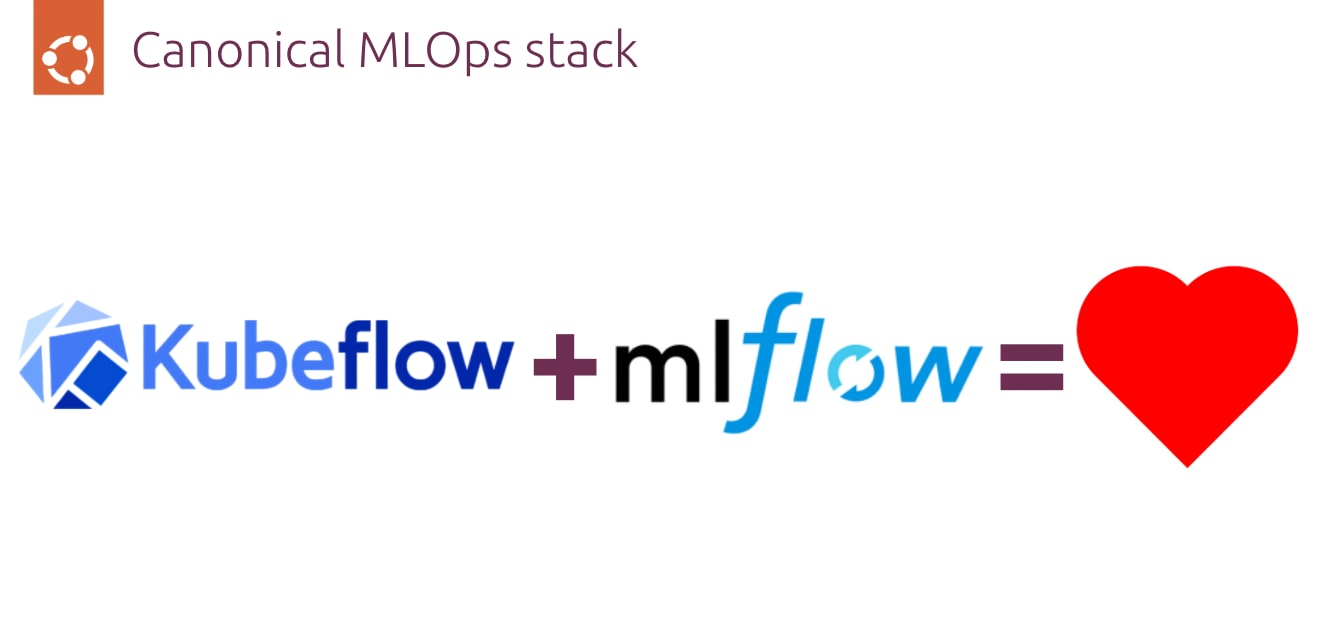
How to choose between Kubeflow and MLFlow?
Choosing between Kubeflow and MLFlow is quite simple once you understand the role of each of them.
MLFlow is recommended to track machine learning models and parameters, or when data scientists or machine learning engineers deploy models into different platforms.
Kubeflow is ideal when you need a pipeline engine to automate some of your workflows. It is a production-grade tool, very good for enterprises looking to scale their AI initiatives and cover the entire machine learning lifecycle within one tool and validate its integrations.
Future of Kubeflow and MLFlow
Kubeflow and MLFlow are two of the most exciting open-source projects in the ML world.
While they have overlapping features, they are best suited for different purposes and they work well when integrated.
Long term, they are very likely going to evolve, with Kubeflow and MLFlow working closely in the upstream community to offer a smooth experience to the end user. MLFlow is going to stay the tool of choice for beginners. With the transition to scaled-up AI initiatives, MLFlow is also going to improve, and we’re likely to see a better-defined journey between the tools. Will they compete with each other head-to-head eventually and fulfil the same needs? Only time will tell.
Start your MLOps journey with Canonical
Canonical has both Charmed Kubeflow and Charmed MLFlow as part of a growing MLOps ecosystem. It offers security patching, upgrades and updates of the stack, as well as a widely integrated set of tools that goes beyond machine learning, including observability capabilities or big data tools. Canonical MLOps stack, you can be tried for free, but we also have enterprise support and managed services. If you need consultancy services, check out our 4 lanes, available in the datasheet.
Learn more about Canonical MLOps
Enterprise AI, simplified
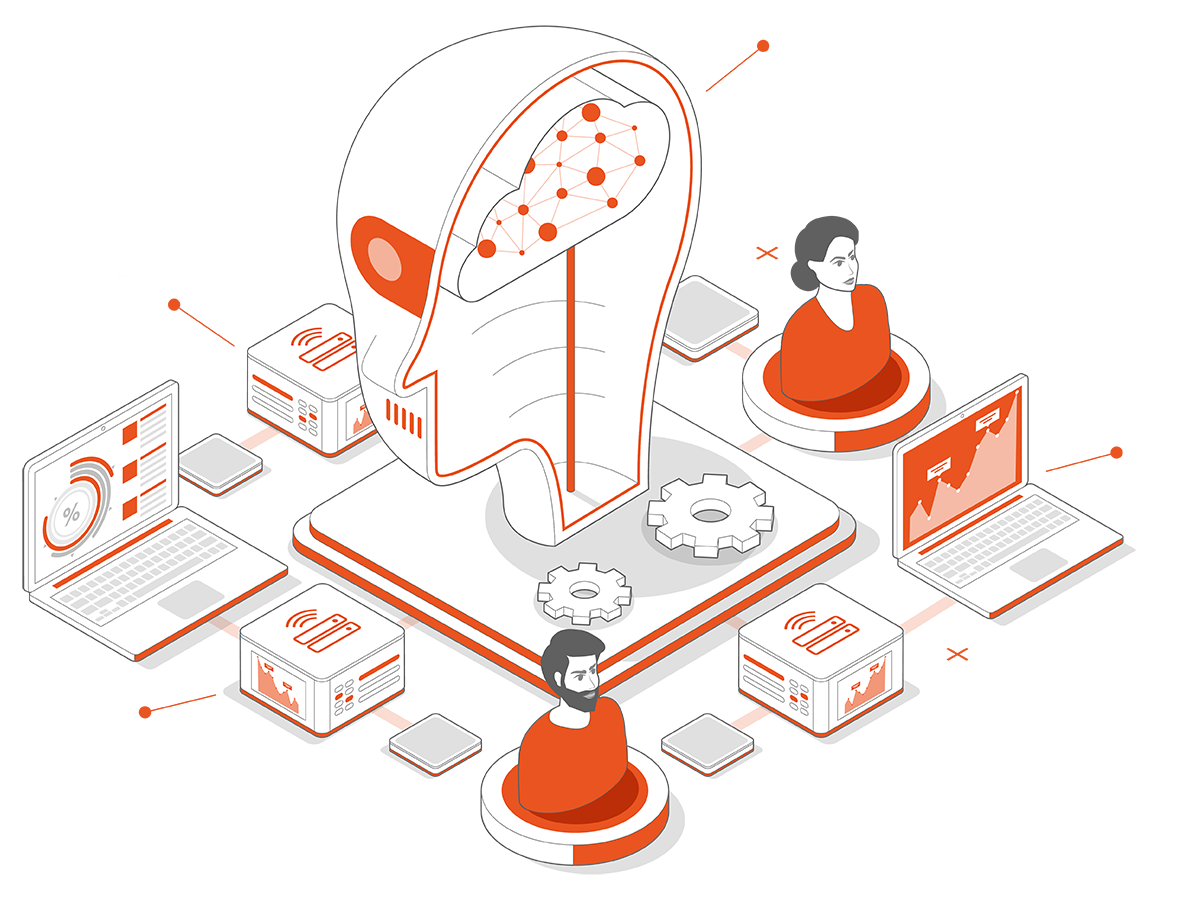
AI doesn’t have to be difficult. Accelerate innovation with an end-to-end stack that delivers all the open source tooling you need for the entire AI/ML lifecycle.